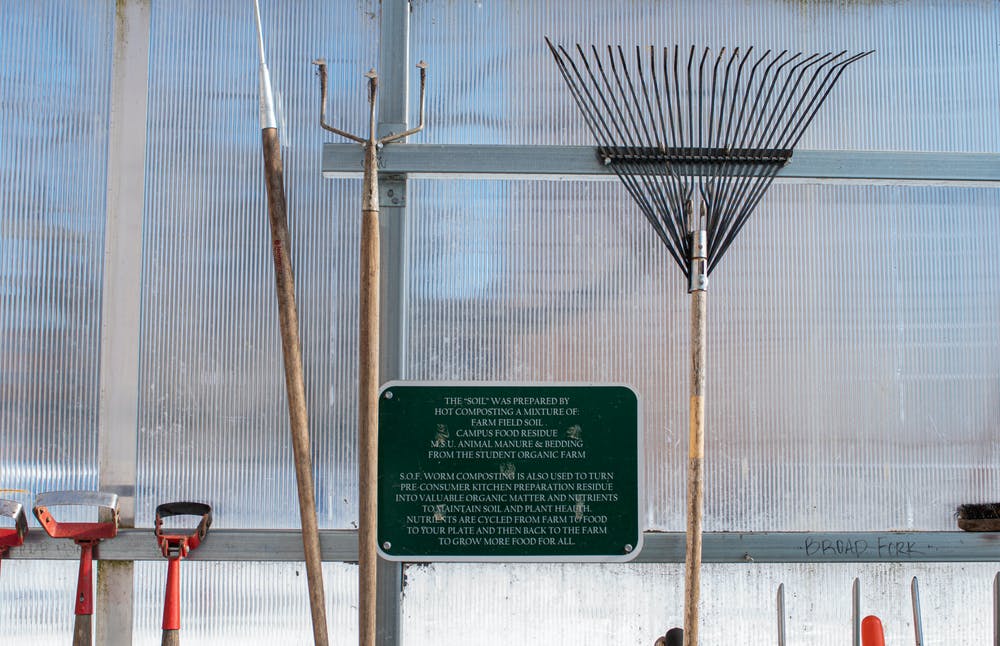
New Michigan State University research found that incorporating in-season water deficit information into remote sensing-based crop models significantly improves corn yield predictions.
The new research, conducted by MSU Foundation Professor in the departments of Earth and Environmental Sciences and Plant, Soil and Microbial Sciences Bruno Basso and his graduate student Guanyuan Shuai, introduces a new and more accurate method to predict crop yields through subfield-level analyses.
Yield predictions allow growers to see what the yields will be across farms before harvesting begins and are important to growers of all backgrounds, from national food supply chains to the individual farmer, as they ensure food security and are the basis for consequential financial decisions.
Traditionally, weather forecasts and previous crop yields are used to predict the next season’s yields, but this method is flawed, Basso said.
“(Previous crop yield prediction) was done at a scale that doesn’t help farmers to make more precise decisions about the forecasting yields of their own fields,” Basso said. “The outlook that is normally provided by either the USDA or other agencies doing it is done at a much larger scale.”
With the ultimate goal of using digital technology, specifically a machine learning model based on remote-sensed imagery and data from a cumulative drop index, to help farmers make more informed decisions, Basso and Shuai began their research by studying 352 fields of varying sizes in Michigan, Indiana, Illinois and Iowa, collecting climate, soil data and more than 2,500 yield maps.
The pair obtained high-resolution digital elevation model, climate and soil data to implement the System Approach to Land Use Sustainability, or SALUS, program, which models crop, nutrient, soil and water conditions each day over many years. SALUS provided the cumulative crop drought index for each field, which highlights the effect in-season water shortages have on crops.
Then, they used Landsat Analysis Ready Dataset products by the United States Geological Survey to calculate the green chlorophyll vegetation index.
They concluded that by including the crop drought index and the green chlorophyll vegetation index in crop yield predictions, predictions could be improved substantially. In fact, their earliest predictions significantly outperformed previous ones.
“We added this information with remote sensing data and we included the predictive models, and we got better prediction results,” Shuai said.
Basso believes that using subfield-level analysis is a promising way to ensure accurate and precise yield predictions and that decisions would be better made off of real-time data as opposed to historical guidelines.
More precise and accurate yield predictions will allow farmers to better manage their resources which will positively affect areas such as the environment, the economy and the national food supply.
“You basically are in a better position to make a decision if you know with a certain level of confidence, which is pretty high level of confidence, that you’re going to be having certain outcomes then, you can manage your decision better,” Basso said.
The findings were published in Remote Sensing of Environment, a leading journal in the field.
“In this research, we are trying to bridge the gaps between the remote sensing area and the agronomical area,” Shuai said. “ We got good comments from our reviewers saying that this has never been done in remote sensing for the last 30 years. We focus on applying our agronomic knowledge and our superior crop models to put all those agronomic information together which did a great job in the remote sensing area. When we combined them together, we got better results.”
The research was supported in part by the U.S. Department of Agriculture’s National Institute of Food and Agriculture, the Great Lakes Bioenergy Research Center, the U.S. Department of Energy’s Office of Science Biological and Environmental Research Program and MSU AgBioResearch.