Descartes Labs uses cutting-edge science to advance global forecasting in areas such as crop production and food security. Their cloud-based computation platform is behind the company’s large-scale analysis, machine learning, environmental change analysis, and global predictions which are used by the commercial, academic, and government sectors.
Descartes Labs is headquartered in Los Alamos, NM, and has additional offices in Santa Fe, San Francisco, and New York City, where you’ll find its supercomputing, machine learning, and image recognition experts working. We had an opportunity to put some questions to Descartes Labs co-founder and Chief Technology Officer, Mike Warren who leads the company’s team of geographers, remote sensing scientists, data scientists, software developers, and applications architects.
Here’s what Mike shared with us:
Descartes Labs just released its new map site that contains three global mosaics: one from Landsat 8, one from Sentinel-1, and one from Sentinel-2a. What is the main purpose of your maps site? Who was it created to help?
These three satellites are generating massive amounts of data that are rich with information about our world. The Descartes Maps site allows the public to access this trove of data in a digestible way, opening up new views of the earth for unique insights. We are demonstrating what these sensors can do today and, through side-by-side comparison of data sets, anticipating what future capabilities will do to unlock greater knowledge about our planet. Our Maps site also showcases the strengths of our platform and the skills of our team, while helping those who study the Earth to imagine other use cases for our geospatial data.
How did you decide on a Landsat 8 R-G-B, Sentinel-2a Red Edge, and Sentinel-1 SAR for your new composites?
We wanted to highlight the unique features of each sensor and demonstrate the flexibility of our underlying platform that was used to create composites from three entirely different systems. Landsat 8 has nearly 4 years of historical imagery, which was enough to produce a 15m (pan-sharpened) cloud-free composite over most of the Earth. Sentinel-2 Red-Edge has nearly equivalent resolution in three bands not present on Landsat 8 that are very useful for determining vegetation health and crop type. Finally, Sentinel-1 is from the entirely different realm of Synthetic Aperture Radar (SAR).
Your data pre-processing chain includes co-registration, map projection standardization, cloud and cloud shadow removal, sensor and sun angle adjustments, sensor calibration and atmospheric corrections. How were the cloud removal and cloud shadow removal algorithms for Landsat 8 developed?
Our pre-processing is described in [the journal article] “Seeing the Earth in the Cloud: Processing one petabyte of satellite imagery in one day” where we demonstrated processing the entire Landsat archive (back to Landsat-4) plus data from MODIS. In total, we processed about a petabyte of data in 16 hours. More recent work explores some of our software infrastructure: “Data-intensive supercomputing in the cloud: global analytics for satellite imagery”. For cloud/shadow removal, we start with the CFMask algorithm from USGS (based on FMask) and then use our proprietary compositing algorithm to eliminate the errors from the initial masked images.
How was the atmospheric correction for Landsat 8 developed?
We used LaSRC (Landsat Surface Reflectance Code) from USGS-EROS. We gratefully acknowledge their efforts and express our appreciation for making that code publicly available via Github. Some modifications were required to obtain pan-sharpened pixels, since that is not an output of LaSRC. Our LaSRC pipeline in Google Compute Engine is capable of processing over 30,000 scenes per hour.
Your pan-sharpened Landsat 8 composite was created using “all imagery collected by Landsat 8,” can you explain that in more detail?
We used all of the Landsat 8 scenes during June, July and August for the Northern Hemisphere and the months of December, January and February for the Southern Hemisphere. Both sets of data were used in the tropics and in areas where monsoon clouds polluted the local summer months. This accounted for about 500,000 scenes. Each scene was approximately 15000 x 15000 pixels after pan-sharpening, but we don’t count the roughly ⅓ of the scene which is black fill. Doing the math, that results in 70 trillion pixels in each of the Red, Green and Blue bands used as input. We also processed the near-infrared band, but did not include it in our released composite.
Using the 70 trillion pixels as input, we collect each observation of the same place on the Earth and turn that into a single pixel which best represents the overall time sequence of pixels. This also involves a map reprojection, since the input is in Universal Transverse Mercator (UTM) and the output is in Web Mercator. Counting up the resulting single layer of Web Mercator pixels results in 3.1 trillion output pixels, which you can see in your browser at the Descartes Lab Maps site.
How would you describe the quality of Landsat 8 data?
Landsat 8 is the jewel of NASA’s Earth Observation program. Landsat 8 provides its greatest value in reliability, calibration and consistent coverage of the Earth’s land area.
Is having open-access global satellite imagery important to Descartes Labs?
The Landsat program was one of the original inspirations for Descartes Labs. We asked ourselves why more people weren’t using this incredible archive of over 40 years of satellite data. We felt that there were tons of interesting problems that could be solved if sensors like Landsat were put into a platform that was optimized for analysis and machine learning. Our original product, a corn production forecast for the United States, was built entirely using open-access satellite imagery from Landsat and MODIS. We only raised a small amount of money and free data helped us to prove our technology without incurring prohibitive data costs.
We are in the business of understanding Earth systems. Our machine learning algorithms cannot create models of these systems without a complete, historical view from above. Unlike drones and other low-flying aircrafts, satellites are able to collect the vast amounts of data needed to view the whole planet on an ongoing basis in real time. This makes the Landsat program a critical and unique resource for us.
Is the continuations of the Landsat data record with Landsat 9 and beyond important?
Absolutely. Having an archive of images of the Earth across time allows the Descartes Labs team to detect and measure changes anywhere in the world and to determine if those changes are short-term, long-term, cyclical, or a one-time occurrence. Ongoing additions to the Landsat archive also allow us to continually train and upgrade our algorithms, such as our agriculture forecasting models, making them smarter and smarter over time. The more years of data we have to train on, the better our models become.
Addendum, March 21, 2017
In early March, Descartes Labs released GeoVisual Search. This is a publicly-available imagery search tool that uses machine learning to identify visually similar objects on massive image mosaics. The initial GeoVisual Search release included imagery from the Landsat global mosaic discussed in this Q&A, as well as a mosaic of China created with Planet’s Dove satellite data, and a high-resolution aerial imagery mosaic of the United States made from data collected by the USDA’s National Agriculture Imagery Program.
With a deluge of Earth observation data coming down the pipeline from both government and industry satellites, Descartes Labs is pioneering news ways to use “computer vision” to find landscape patterns and to better understand Earth system processes. Ryan Keisler, head of machine learning at Descartes Labs, told us the next steps for GeoVisual Search will be to add and amass more large scale geospatial datasets and to hone their deep learning algorithms. Keisler, who worked as a research astronomer for years, has turned his gaze Earthward and is keen to see what secrets about our home planet his AI search tools can uncover when coupled with Earth observation data.
Further Information:
+ Using Petabytes of Pixels with Python to Create 3 New Images of the Earth, Descartes Labs
addendum links
+ Search Earth with AI eyes via powerful satellite image tool, C|NET
+ Ever Want Image Search For Google Earth? This AI-Driven Tool Does That, Fast Company
+ Why this company built a search engine for satellite imagery, CBC
Any use of trade, firm, or product names is for descriptive purposes only and does not imply endorsement by the U.S. Government.
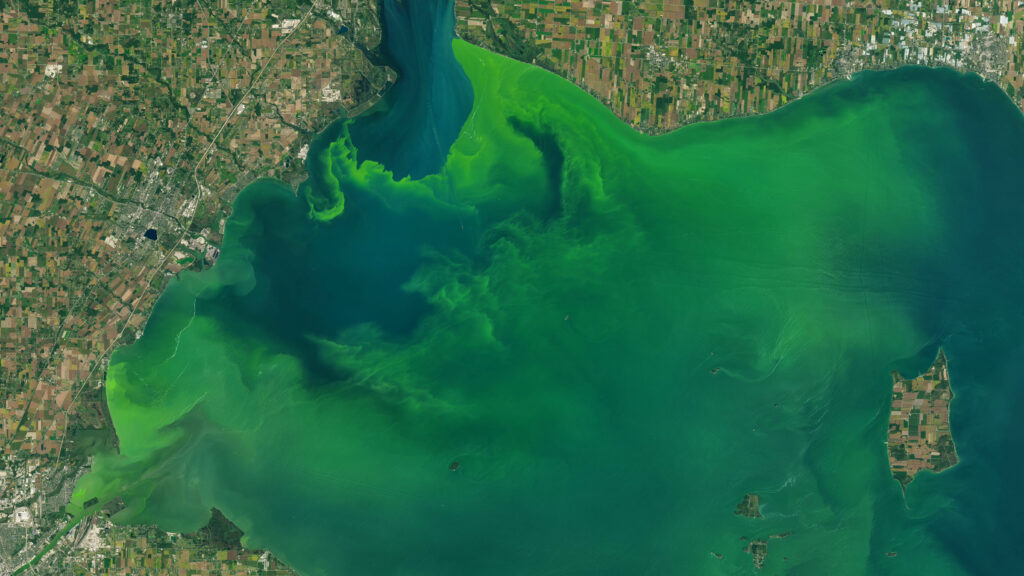
Be Part of What’s Next: Emerging Applications of Landsat at AGU24
Anyone making innovative use of Landsat data to meet societal needs today and during coming decades is encouraged to submit and abstract for the upcoming “Emerging Science Applications of Landsat” session at AGU24.