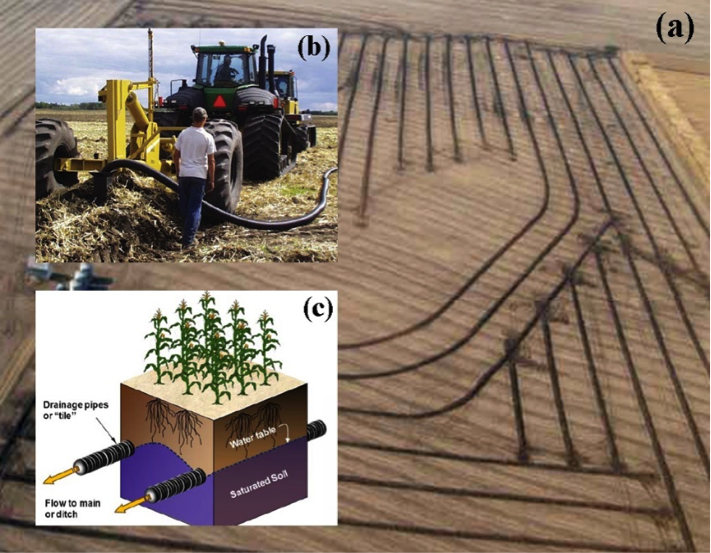
Mr. Eunsang Cho is a Ph.D. candidate at the University of New Hampshire working with Dr. Jennifer Jacobs. Here is what Eunsang shared with us about the work he is presenting today at #AGU18:
Mapping Agricultural Tile Drainage Expansion from Satellite-based Vegetation and Thermal Data Using Google Earth Engine Machine Learning
What are the major findings of this research?
We are able to produce annual high-resolution (30 m) agricultural subsurface drainage maps (known as tile drainage (TD) maps) for the Northern Great Plains regions of the U.S. using multiple satellite and model datasets via Google Earth Engine’s cloud computing platform. Soil properties, climate variables, and land surface temperature are the strongest predictors of tile drainage installation.
What are the implications of your findings?
Operational satellite data and machine learning provide a powerful TD detection technique that can be used to greatly improve water resource management and flood forecasting.
What insight did you gain from Landsat that would have been difficult to ascertain otherwise?
The high-resolution (30 m) of Landsat-based vegetation and thermal products—including the normalized difference vegetation index (NDVI), the normalized difference Water index (NDWI), the enhanced vegetation index (EVI), the greenness index (GI) and shortwave infrared reflectance—develop high-resolution TD maps. These maps clearly show within field TD effects on the soil water and temperature.
Will the drainage maps you developed be used by NWS flood forecasting system?
Currently the maps are produced for a limited extent that is of value to the NOAA/NWS North Central River Forecasting Center (NCRFC). In the upcoming year, we will work with their forecasters to determine the best approach for using the maps in their flood forecasting system. At the same time, UNH researchers are working the NASA’s GSFC Hydrological Sciences researchers to include the TD maps into NASA’s Land Information System (LIS). Successful integration will enable the evaluation of hydrological changes by recent TD expansion using the multiple land surface models (e.g. Noah, VIC, and NASA catchment model etc.) that are available in LIS. We expect that the maps will improve the capability of hydrological modeling and flood prediction in tile drainage dominated regions.
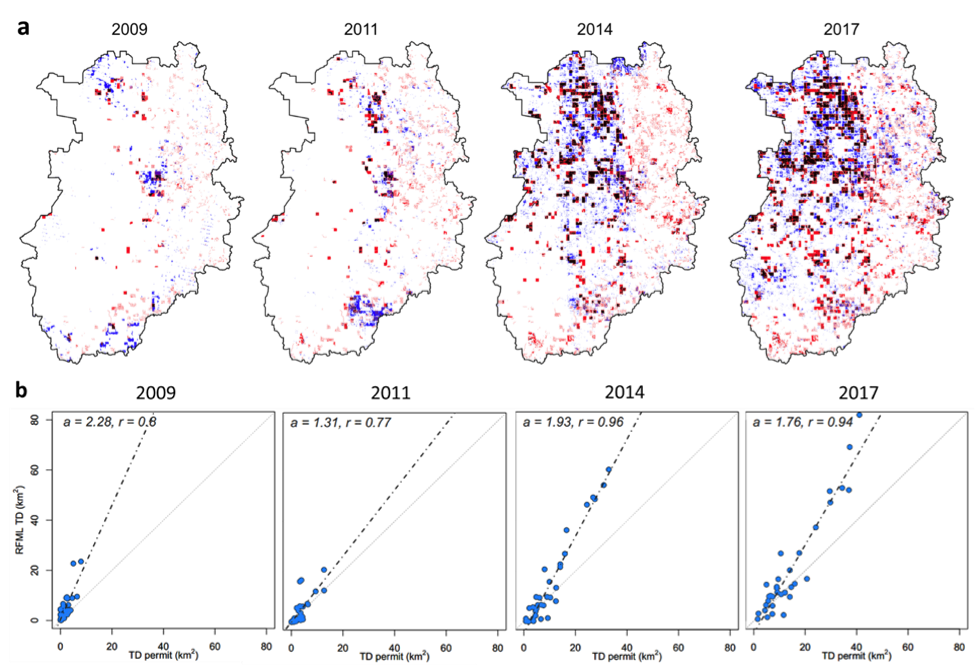
Co-authors:
Jennifer M Jacobs
University of New Hampshire
Xinhua Jia
North Dakota State University
Simon Kaartz
University of New Hampshire
This work was supported by the NASA Water Resources Applied Sciences Program (NNX15AC47G)
Anyone can freely download Landsat data from the USGS EarthExploreror LandsatLook.
Further Reading:
+ Landsat at #AGU18