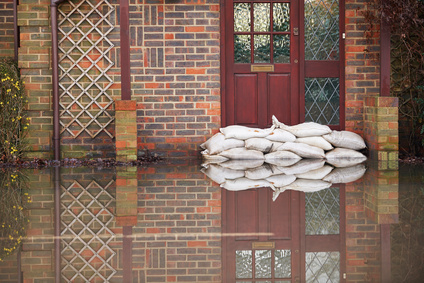
By Laura E.P. Rocchio
This afternoon at #AGU16, Beth Tellman, a PhD Candidate in Geographical Sciences and Urban Planning at Arizona State University and a co-founder of Cloud to Street, gave a talk on her work developing the largest database of historic flood maps. Here is what she shared with us about this work:
Presentation Title
Developing a Global Database of Historic Flood Events to Support Machine Learning Flood Prediction in Google Earth Engine
What are the major findings of this research?
Using Google Earth Engine, Cloud to Street and our partners at the Dartmouth Flood Observatory (DFO) are developing the largest database of spatial extent for historic floods, specifically to over 5,000 floods recorded since 1980 by the DFO. The results, to be released as an open database in the summer of 2017, will first contribute to the understanding of historical flooding, how these hazards have changed over time at a scale and resolution never before possible. Adapting and improving existing remote sensing flood detection algorithms for high capacity cloud computing via Google Earth Engine and applying them to decades of Landsat, MODIS and Sentinel imagery, dramatically improves our estimates of the historical flood footprint, especially in urban areas. The temporal advantages of satellites like MODIS, with the spatial resolution of satellites like Landsat, help us get a more realistic sense of the population in the floodplain in historic events. This spatial history can then be used to train machine learning models to predict the full flood plain as well as future flooding.
What are the implications of your findings?
According the UN, 250 million people are affected by flooding each year, more than any other hazard, and the number of people and GDP exposed flooding is expected to double by 2030 due to climate change and population migration. Accurate information about past floods and future risk is critical as cities, national governments and other users prepare. Our database of mapped historic floods will address the information gap in flood risk information by scaling remotely sensed flood detection for global and continuous implementation for scientists and emergency managers alike.
Dartmouth Flood Observatory (DFO) has recorded nearly 5,000 flood events since 1980 but only approximately 5% of these floods have been mapped, typically using MODIS imagery. We’ve developed algorithms in Google Earth Engine to map floods since 2000 with MODIS, and when available from 1984- present using Landsat and 2014-present using Sentinel. Our methods can also be applied to other national level flood country databases. For example, the NCDC (National Climate Data Center) in the USA has recorded over 10,000 riverine flood events at the county level since 1950. In addition, we’re starting to develop approaches to scrape publicly available news articles that could expand these available databases procured by DFO and NCDC. Where the satellite record aligns with these databases we’ll be able to add spatial detail with our flood detection algorithms.
Furthermore, developing a spatial flood history is important information to calibrate and train flood models (both physically based and machine-learning oriented), understand risk and probability for insurance companies and analyze the changing pattern of flood vulnerability in a watershed. We are already leveraging the spatial flood history of Senegal to do flood vulnerability analysis for Senegal in conjunction with the French Development Bank, where initial pilot studies have identified close to 300,000 local people at high physical and social risk to inland flooding.
What insight did you gain from Landsat that would have been impossible to glean otherwise?
The resolution of Landsat imagery and the size of the Landsat database enables critical insight for scalable, high resolution flood detection in several key ways. First, in watersheds where we happen to have Landsat imagery during an event, we can estimate the flooded area at a higher resolution of 30m instead of 250m, which is what we get using MODIS. This increased resolution is particularly critical in urban areas, where flooding is very concentrated and not resolvable at 250m because of high albedo urban infrastructure or because the flooding tends to be concentrated in narrow corridors along streets. Second, Landsat’s history extends 15 years previous to MODIS, expanding the history of the database.
In our pilot study in Senegal, we analyzed the spatial flood history for 6 major floods since 2001, using 837 MODIS images and 266 Landsat images. For 4 of those floods, relatively cloud-free Landsat imagery was available. In these three floods, our water detection algorithms using MODIS detected a total of 2,198 km2 of inundated area, while our water detection algorithms using Landsat detected nearly double that amount – 4,501 km2. Even more important, much of the addition in flooded area was in urban areas like Dakar and Saint Louis- where MODIS detected only small areas of inundation. For the three floods where both Landsat and MODIS imagery was available, the total population exposed MODIS flood detection was estimated at only 48,000, whereas with Landsat flood detection, we estimated 472,288 people exposed to flooding. This is nearly an order of magnitude difference due the limitation of MODIS.
How and by whom do you foresee your historic flood map database available in the Google Earth Engine Catalogue being used?
We expect the global flood map database to be useful to scientists, national and international governments, relief agencies, insurance companies, and civil society organizations. This database can help these audiences understand historic flood histories, examine how trends in land use, climate change, and infrastructure may influence flood damages, train new machine learning approaches to for flood prediction, and many more applications.
For instance, one researcher on our team used flood imagery in his local hometown of Boulder, CO to convince the community to reject a public housing development project that was in a floodplain (by showing a recent flood in the image he sent to his community members). He was successful in rallying his community to reject the project. Historic flood imagery can provide critical information about who is at risk, to develop better emergency plans, and prevent public and private investment in infrastructure in places where it is likely unviable.
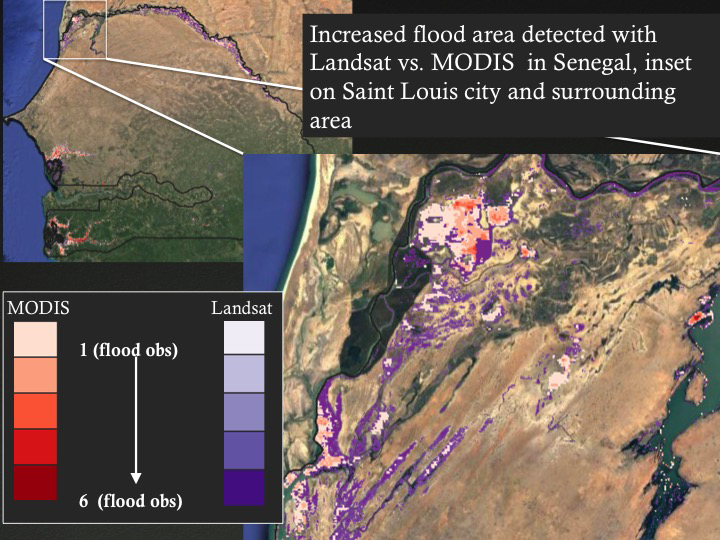
Co-authors:
Jonathan Sullivan
University of Michigan
Albert Kettner
University of Colorado at Boulder
G Robert Brakenridge
University of Colorado at Boulder
Daniel A Slayback
Science Systems and Applications, Inc.
Catherine Kuhn
University of Washington Seattle Campus
Colin Doyle
University of Texas at Austin
This work was funded by a Google Earth Engine Research Award in Collaboration with the Dartmouth Flood Observatory at the University of Colorado Boulder. The pilot study presented for Senegal was funded by the French Development Bank.
Anyone can freely download Landsat data from the USGS EarthExplorer or LandsatLook.
Further Reading:
+ Landsat Abounds at #AGU16